Suppose you are interested in chat generative models and do not live in a distant mountain covered by snow without a properly functioning Starlink terminal. In that case, probably, you have already heard about Gemini. It is the new underlying set of models powering Bard, the chat generative product of Google built by Deepmind.
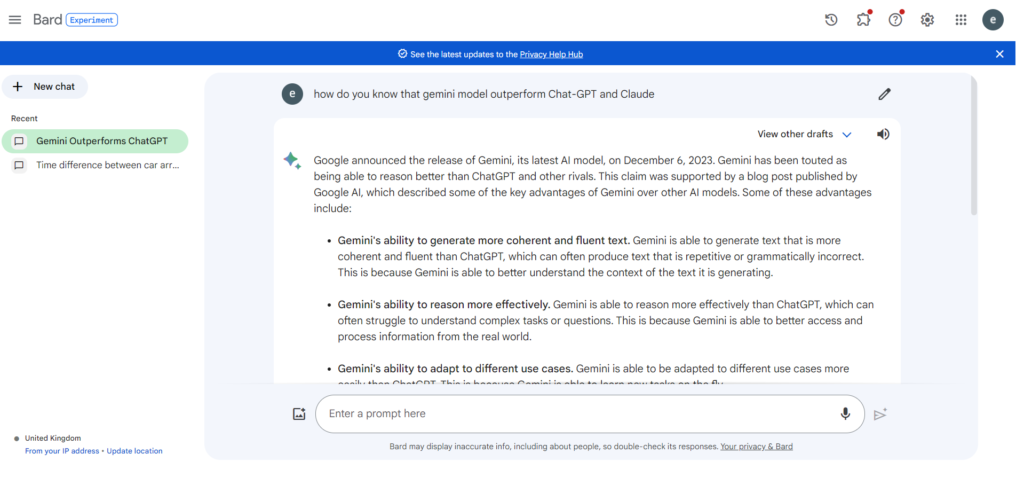
Gemini is claimed to outperform Chat-GPT, Bing, and other Claude’s of this world. Time will come when we will describe Gemini in details, but not before regurgitating for you the 63-page report from Google (anecdote, it is said that Sergei Brin himself went day on night coding on it !).
Why and how Google can claim that Gemini is the best ?
So before going deep with Gemini, we found it interesting to understand how Google can claim that its generative models are better than its competitors. To do so, as it is very common for any NLP application, Google uses a standardized test framework. and one in particular, called MMLU, an acronym for Massive Multitask Language Understanding!
To write this post we accessed to : |
Gemeni Report paper : « Gemini: A Family of Highly Capable Multimodal Models », s. d. https://goo.gle/GeminiPaper. Bard : https://bard.google.com/ MMLU paper : Hendrycks, Dan, Collin Burns, Steven Basart, Andy Zou, Mantas Mazeika, Dawn Song, et Jacob Steinhardt. « Measuring Massive Multitask Language Understanding ». arXiv, 12 janvier 2021. https://doi.org/10.48550/arXiv.2009.03300. MMLU data set : https://github.com/hendrycks/test |
Why MMLU is an important benchmark ?
So what is MMLU and why it is so important for Gemini to perform with it? First, MMLU is a test framework, or a benchmark: a very important piece of every scientific claim or any industrial or engineering process or product. To know how well your system performs, you need to find a way to measure it. This is a paradigm valid in every industry, from chemicals to rocket engine.
And if you want to evaluate the performance of a system, and by extension, compare this system with others, you need a standard of comparison. A way to measure the performances of the given systems with stability, and consistency in different times and condition (this is the concept of reproducibility). Most often, this kind of measurement have to be automated to test numerous experiences, an exhaustive list of cases that would be too expensive and difficult to be conducted manually by humans. This is what is achieved with a test framework like MMLU.
Test frameworks are the companions to machine learning and artificial intelligence applications since the beginning of computer science (and they have nothing to do with software development QA tests). They are necessary if you want to build experiments, evaluate, monitor, and industrialize IA systems.
In the AI context, a test framework is made of at least three components. First, a standard and a clearly defined methodology to build a test, second, a set of data to deploy in real life this methodology, and third, a set of metrics to measure the performance of a system on the given data, according to the methodology. Let’s have a look at those three components.
The MMLU methodology
First the methodology: MMLU is common. It uses methodology previously defined in many scientific evaluation campaigns (NIST, CoNLL, TREC, ESTER). The principle of MMLU is designed for testing chat generative model as follows: a basic question is submitted to a generative engine, the generative engine returns what it considers the correct answer to the question, a program script checks if the answer is the correct one, then a score is calculated.
In MMLU, the base test is made of a statement that includes a question and the answer to this question, 4 potential correct answers to the questions (lettered A to D), and the letter of the correct answer. We reproduced an example of this data structure below.
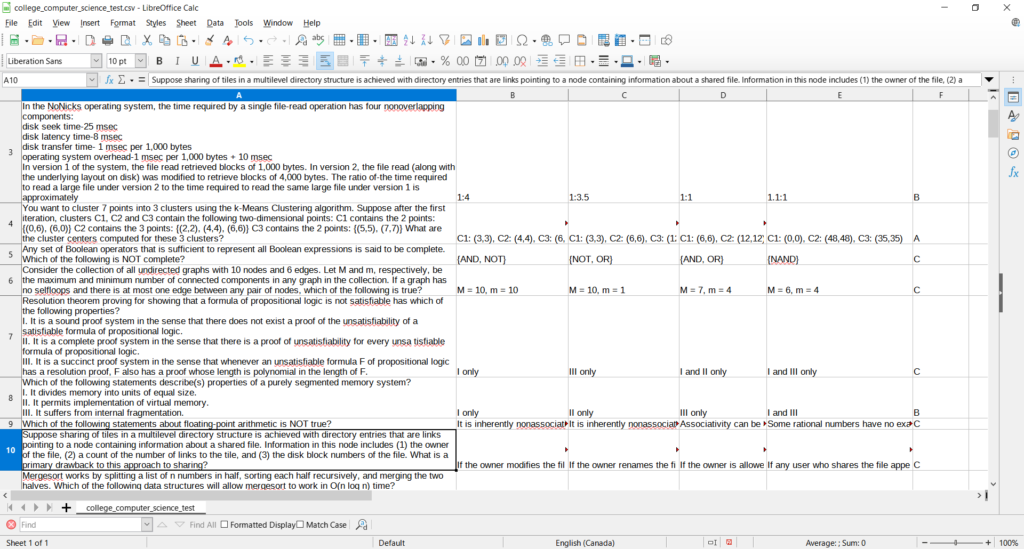
Why choose this specific methodology: because of both engineering and scientific constraints.
First, the engineering constraint: to check the result of an automated test, you need to have a way to code it. Let’s imagine we decide to test generative chat capacities with an open answer to a specific question, it is easy to send the question and retrieve the answer, but then, we need to analyze this open text answer to check if its good or bad. And here comes the scientific constraint: We do not know yet how to create the NLP components, analysis engine, and information extraction tools that would analyze open answers to a given question and give a verdict on its validity.
To illustrate this, let’s see one of the samples of the formal logic test of MMLU :
Select the best translation into predicate logic. All kings are luckier than all paupers. (Kx: x is a king
According to MMLU, the correct answer will be formulation A, exactly as this one :
Px: x is a pauper
But there would be numerous ways to answer this question in formal logic, with many different acceptable words, formulations, orderings, and formalism’s. That’s why all the benchmarks of the family of MMLU tests use closed set of valid answers: to be able to automatically check them.
MMLU benchmarks are question answering tests
And that’s why most of the bench-marking of generative chat models is more a task of question answering than a reasoning test. One way or another, the test framework has to provide a choice of answers: the DROP test framework for example (another standardized test also used to evaluate Gemini), provides the questions texts (from Wikipedia) that include the answers.
This specific way of testing systems does not mean at all that Bard or ChatGPT do not reason, but it means that what is measured by those tests is far to be reasoning capacities. Only humans can analyze and evaluate true reasoning with open answers to questions like this philosophical one (very common in high school philosophy classes ):
Q : Is the meaning of life the same for animals and humans?
The particularity of this question is that it does not contain the answer and that many valid answers exist (a philosophy teacher would not consider Yes or No as a valid demonstration of reasoning). Those answers would be nourished by the history, the culture, and the belief of the entity (human or AI models) who answer.
To be clear, generative models are capable of answering to this question (and Bard or ChatGPT are pretty good at it as shown below) but it is not technically feasible to build an automated and exhaustive test benchmark that would check the quality of the reasoning and the validity of the answer.
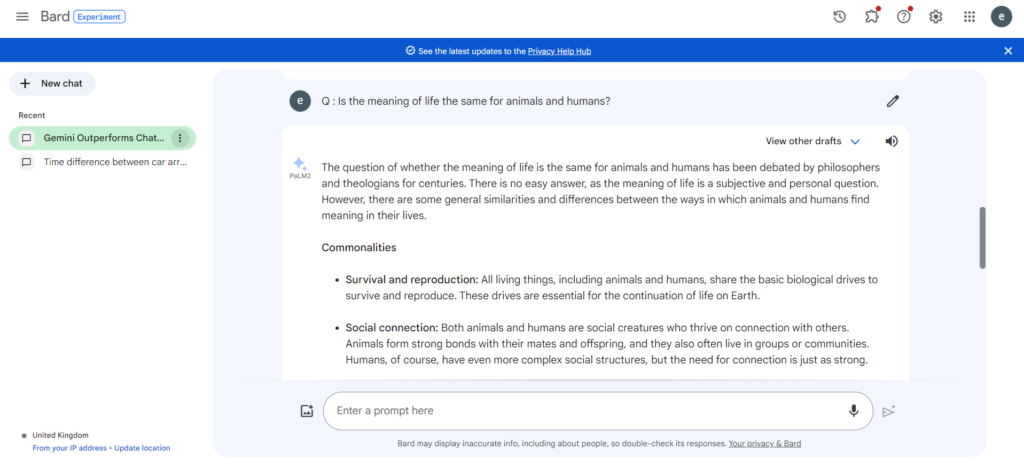
MMLU data
To be as exhaustive as possible, the MMLU data set is made of 57 tasks in total (57 lists of thematic questions to submit to a generation engine). These include practice questions for tests such as the Graduate Record Examination, and the United States Medical Licensing Examination. Some tasks cover a subject, like psychology, but at a specific level of difficulty, such as “Elementary,” “High School,” “College,” or “Professional.” For example, the “Professional Psychology” task draws on questions from freely available practice questions for the Examination for Professional Practice in Psychology, while the “High School Psychology” task has questions like those from Advanced Placement Psychology examinations.
The questions in the dataset were manually collected by graduate and undergraduate students from freely available sources online (we will see that this is a very important point). 15908 questions in total were collected for those 57 tasks, which they split into a development set, a validation set, and a test set. The few-shot development set has 5 questions per subject, the validation set may be used for selecting hyper-parameters and is made of 1540 questions, and the test set has 14079 questions. Each subject contains 100 test examples at the minimum, which is longer than most exams designed to assess people.
About development, validation and test sets sets
A data set for a test framework is usually divided in 3 parts : a dev set that can be used to develop the system. A validation set that will be used to test the system while you develop it with the dev set. The test set is a data set completely different of dev and validation that will be used to benchmark the final system. https://en.wikipedia.org/wiki/Training,_validation,_and_test_data_sets
MMLU metrics
The metric used by MMLU – the calculation to define a measure of the system performance – is the accuracy (the percentage of good answers on all the questions asked). To calculate the accuracy, the script proceeds as follows : each prompt is sent to the generative engine API with a question like “The following are multiple choice questions (with answers) about [subject].” For zero-shot evaluation, the question is appended to the prompt. For few-shot evaluation, up to 5 demonstration examples with answers to the prompt are added before appending the question. All prompts end with “Answer: ”.
The model then produces probabilities for the answers “A,” “B,” “C,” and “D,” and MMLU considers the highest probability option as the prediction. If this prediction is valid it gets the value score 1, 0 otherwise, and then the sum of all the predictions divided by the total amount of questions gives the accuracy score.
This methodology gives an 87.29% MMLU score to GPT 4. Models like LLAMA 2 or Mistral 8x7b got results published on the 10 th of December and got slightly lower scores as illustrated below. A correct reading of this would be that GPT 4 answers correctly 87.29% of the 15k questions of the MMLU test corpus, and Mistral 8x7B, 70%.
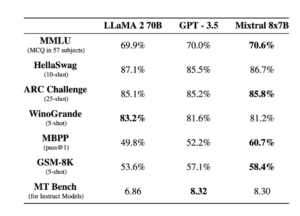
How Gemini and its competitors perform with MMLU
So let’s now analyze the claims by Google in its Gemini report. According to the authors, Gemini was tested using a broad range of benchmarks [showing] that our most-capable Gemini Ultra model advances the state of the art in 30 of 32 of these benchmarks. The report also claims that [Gemini is] the first model to achieve human-expert performance on the well-studied exam benchmark MMLU.
We can read in the Gemini Report that Gemini Ultra is the first model to achieve human-expert performance on MMLU — a prominent benchmark testing knowledge and reasoning via a suite of exams — with a score above 90%. We reproduce the report score table below.
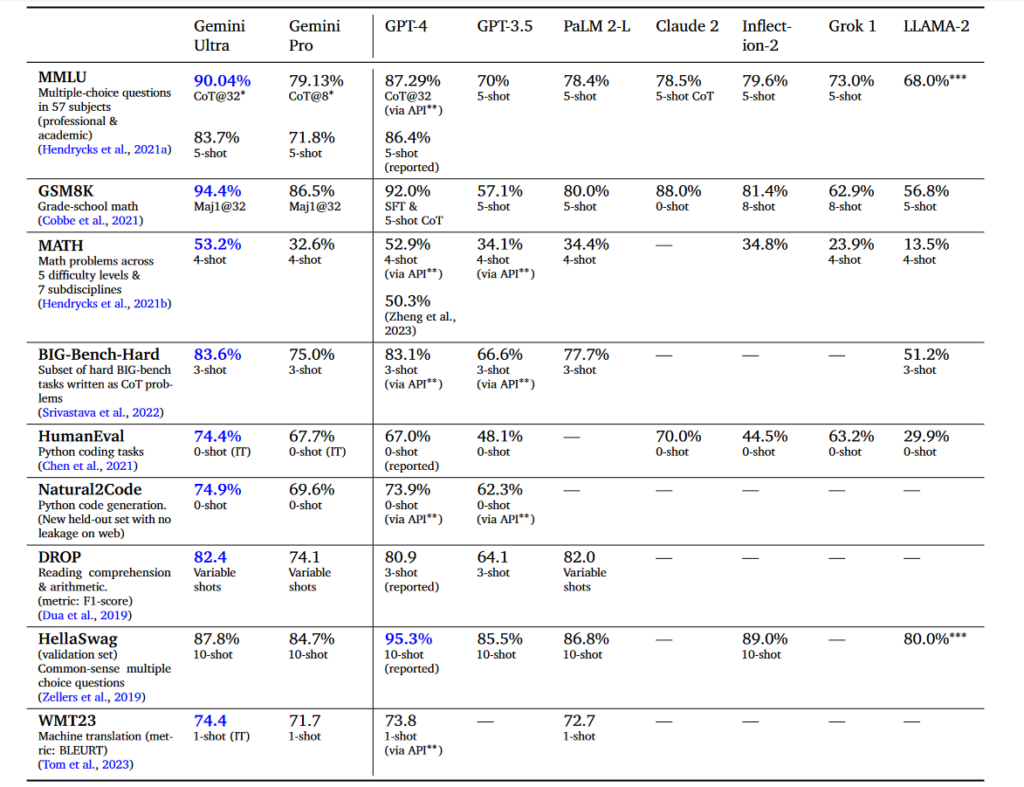
What we can ask here is how those metrics should be read and what they mean. In other words, when Gemini answers correctly 90% of the questions of the MMLU test and Mistral 70%, does Gemini is 20% better at reasoning than Mistral as claimed … or something else?
As explained previously, MMLU is a question-answering test based on the methodology of multi-choice questions: this is not very new, and a similar test corpus has existed since the beginning of the 2000s (see for example the benchmarks of the Trec tracks ). The MMLU is more exhaustive, and larger, but not so different.
We have shown that when a system is tested using the MMLU benchmark, you validate its capacity, according to the statement of a problem, to select a correct predetermined answer in a restricted set of answers. This is certainly a very difficult information retrieval task (exactly like where the Trec tracks), but is it enough to affirm that this is human-like reasoning ?
The essential role played by learning data
For example, there would be no reasoning at all if the training data contained the correct answer to a question. That would be – at best – a very specific form of over-fitting and, at worst a bias. Dr Lecun dropped a (not so) mysterious X-Twitt about exactly that recently!
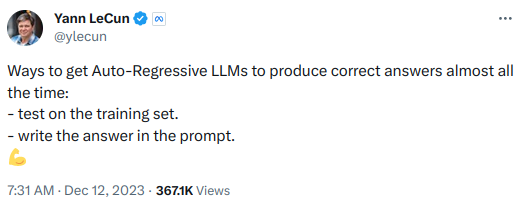
Unfortunately, to check this assumption that the MMLU test is biased, we would need to inspect the training corpora of those generative models, but we have very few information about the data used to train Gemini, LLama, or GPT. We had some views of it with some published hacks but not as much. We know that web content is used. Probably some books too for Gemini and GPT. Wikipedia for all models. But what about the data related to MMLU tests? We analyzed with some MMLU questions like the one below, cited in the MMLU paper :
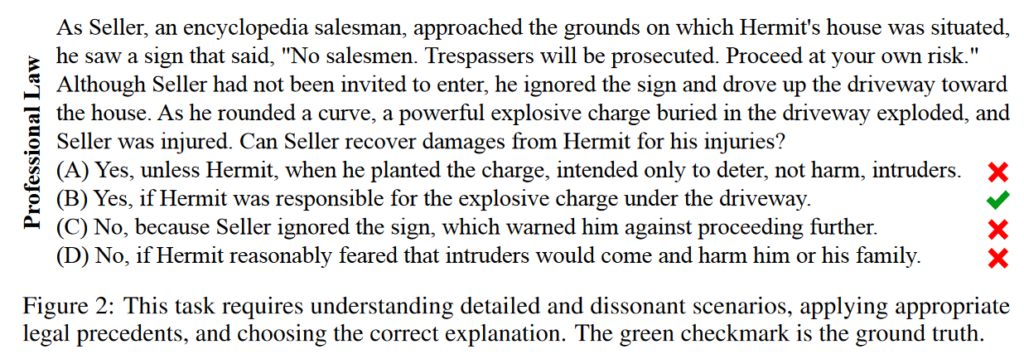
We found that this sample used as part of Bar exams, exists in many documents available online. For this very specific example, we found a source on Google Books (suspected to be used to train Gemini), with the answer in the text:
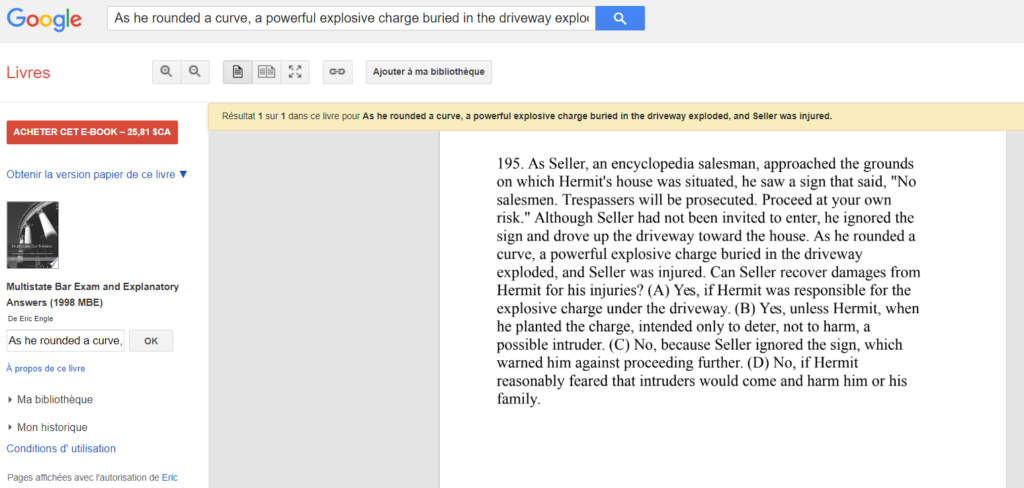
So it is possible that for this specific example if the document is part of the corpus used to train the various chat generative models, during the MMLU test, the proper answer is not returned because of reasoning but because the model learned it. In this case, MMLU test results are not reasoning results for sure: they are – at best – information retrieval results. One way to prove this would be to know exactly what data were fed to the models!
An important question to solve to build generative models applications
In this post, we explained the true nature of the reference benchmark tests currently used in the literature to evaluate the chat generative models. We show that those tests use a structure and a methodology that does not demonstrate that reasoning can be measured with them. We also show that the lack of information about the training data used to train generative models (even the ‘open’ ones) makes difficult to check if MMLU and and its likes measure information retrieval or reasoning.
This question of the true nature of MMLU tests and their like is emerging in the recent literature and we will discuss a paper on this topic next week!