It’s a little provocative title. Not mine! It is the title of a very serious pre-print recently published on ArXiv by highly competent scientists with the explicit title: Don’t make your LLM an evaluation benchmark cheater!”
What is this about? As you can imagine, it is about benchmarking the performances of LLMs, and more specifically about not making it in a way that could be biased. The authors study an exciting concept related to LLMs training and benchmarking: benchmark leakage. Moreover, they conducted numerous and exciting experiments to evaluate how much the measured performance of LLMs using a test benchmark like MMLU is influenced by the presence of MMLU data in the pre-training phase of LLMs.
Training models to check the influence of data leakage
Before going into the details, a quick reminder on how LLM and generative tools are trained, as most of the paper experiments are made possible because the authors – to make their point and show their theory – completely trained some open source LLM models from scratch.
As a reminder, an LLM is built through multiple phases. The most important ones are pre-training and fine-tuning,
The first one (and most complex one in terms of computing power) is the pre-training which takes a long time – a few days to a few months – to complete. With auto-regressive models (eg. GPT, BARD), which are uni-directional and are trained to predict the next word without seeing the succeeding ones (because those models are specifically optimized for better language generation), during the pre-training process, we are not training the model for specific language tasks (like a generation or named entities recognition) but only to make it learn how to predict words in a sentence. This pre-training process builds the pre-trained language models (PLM). It is usually costly to train PLM (a few thousand to more than a million dollars) making the experiments presented by the papers we describe here very ambitious.
To write this post we used / pour écrire cet article, nous avons consulté: |
Zhou, Kun, Yutao Zhu, Zhipeng Chen, Wentong Chen, Wayne Xin Zhao, Xu Chen, Yankai Lin, Ji-Rong Wen, et Jiawei Han. « Don’t Make Your LLM an Evaluation Benchmark Cheater ». arXiv, 3 november 2023. https://doi.org/10.48550/arXiv.2311.01964. |
After the pre-training, the fine tuning process
During the fine-tuning process, a task-specific layer (eg. sentence classification, named entity recognition, question-answering, etc) is added to the PLMs and carries out the usual backpropagation method using a suitable loss function. Reinforcement Learning from Human Feedback (RLHF) is the method of fine-tuning using samples of prompts (prototype of question and answering corrected by humans for a bot) for GPT or Claude generative models.
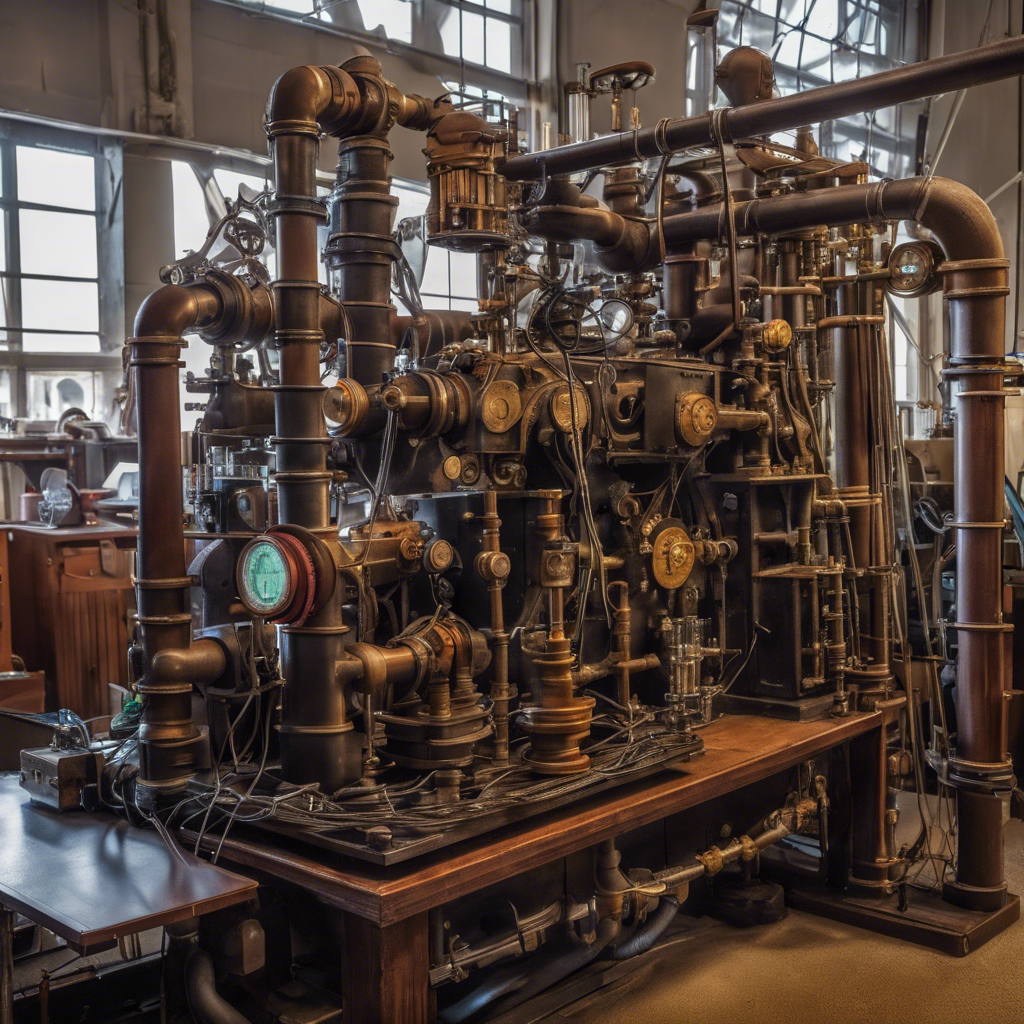
The question of data leakage during training
The issue is that if during any phase (and most specifically the pre-training phase), part of the training data used includes the answers to the questions asked by normalized test benchmarks, those benchmarks are biased.
By biased we mean that the benchmark might not measure what it claims to do (like reasoning for MMLU for example) as we explained in this post because the model has already seen the answer to the question during the training process.
And “To make matters worse, the detailed composition (e.g., data sources) of the training corpus is often regarded as the core “secret” of existing LLMs. Therefore, it becomes difficult to directly examine the contamination issues when evaluating benchmark maintainers.” (Zhou et al., 2023, p. 2)
Such leakage has already been demonstrated in multiple instances: it has been shown that GPT-3 included the Children’s Book Test dataset (an other test benchmark) in its pretraining corpus (Hill et al., 2016), and LLaMA-2 authors has mentioned that the contexts in the BoolQ dataset (Clark et al., 2019) are extracted verbatim from the webpages, which may be included in the publicly available corpus. We also have shown in our previous articles that some Bar exams used in the MMLU benchmark are available on line (with answers) and could have been used to train Gemini (the Chat LLM from Google).
Demonstrating how leakage can boost benchmark results
So it is known that benchmark data can be leaked in training data, but we do not know how much (because of the secrecy of the data set used) and as we do not know the size and the nature of the potential leakages it is difficult to evaluate their potential impact. Here come our authors who do not answer the question of the volume of the leaks but, built an experiment that will allow us to know what would be the impact of a leak.
To make this empirical study, they selected the MMLU benchmark (frequently claimed to be a reasoning test and reading comprehension test) for evaluation. That is particularly interesting for us as it is precisely this MMLU benchmark that we challenged in our last post.
What they did then was ambitious : they retrained from scratch four real open source models (by real, we mean models where we have both the code and the training data publicly available) in five different configurations, with and without a leak of MMLU benchmark data. Don’t know where they found the money to conduct such experiments but they did it! They trained :
- GPT-Neo-1.3B (Black et al., 2021): it is a Transformer-based model with GPT-3 architecture, pre-trained on the Pile (Gao et al., 2021) dataset. •
- phi-1.5 (Li et al., 2023): it is a 1.3B model trained on “textbook quality” data of ≈27B tokens, and can achieve comparable performance as much larger models. •
- OpenLLaMA-3B (Geng and Liu, 2023): it is an open-source project to reproduce LLaMA model with a permissive license, pre-trained on RedPajama dataset (Computer, 2023) of over 1.2T tokens.
- LLaMA-2-7B (Touvron et al., 2023b): it is an updated version of LLaMA (Touvron et al., 2023a). It has been pre-trained on a mixture of publicly available online data of 2T tokens.
As you can see, they also retrained LLaMA-2 and I am still puzzled by this as training data for this model are not documented as far as I know (the paper should be more detailed on this point). And the five configurations were as follows:
- Model with original training data
- Model with original train data and MMLU training data
- Model with original train data, and all others tests training data
- Model with original train data, all others tests training data and their tests data
- A fifth configuration is tested that authors suggest to not consider at this time for experimental reason.
Then all those models are tested with 8 benchmarks, and the results are below. We will not comment on all the results in detail (we suggest our reader to deep dive into the paper for that). We only focus on MMLU and we see, with no doubt, that when you include the answers to the tests in the training data, MMLU performs better in reasoning tasks!
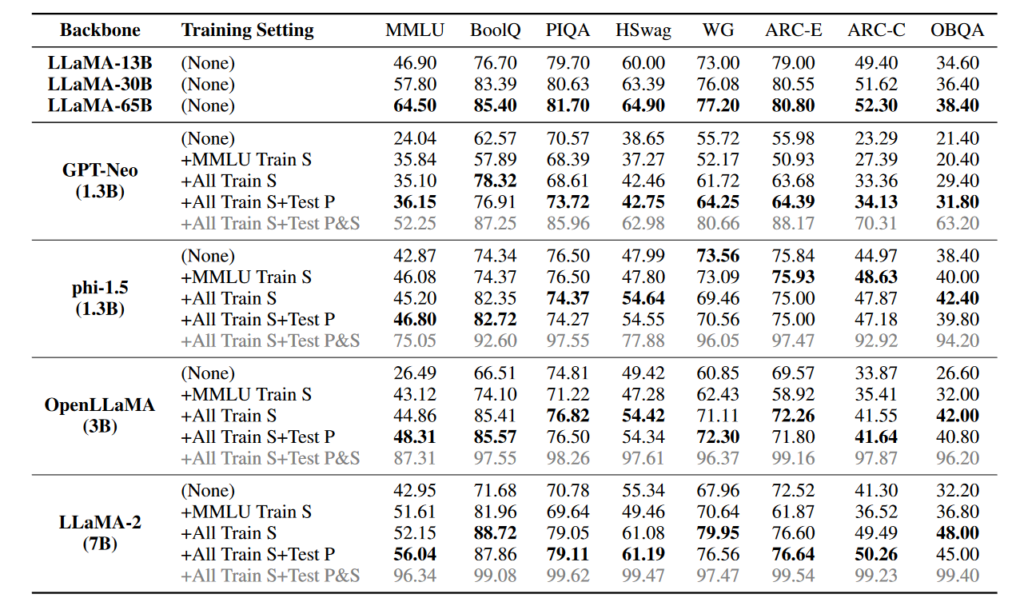
As the authors state : the experimental results reveal that benchmark leakage can lead to an unfair boost in the evaluation performance of LLMs. Smaller LLMs (e.g., a 1.3B model) can be deliberately elevated to outperform 10× larger models on certain tasks. As a side effect, the performance of these specially trained LLMs on other normally tested tasks would likely be adversely affected if we fine-tune or train the model only with these leaked data.
Some recommendations for LLM developers
As said previously, this work does not prove that benchmark data (with questions and answers) are used to train big names of LLMs. But it gives a very good idea of what would happen if it was the case. And that leads to some recommendations for LLM practitioners.
To improve the use of existing evaluation benchmarks, the authors present several guidelines for both LLM developers and benchmark maintainers. They hope this work can draw attention to the need for better training and evaluation of LLMs. I would add that it, is very important, especially for industries that will try to deploy in real-world applications and would have difficulties understanding why their implementation would not perform according to the benchmarks published by the vendors of the LLMs APIs!